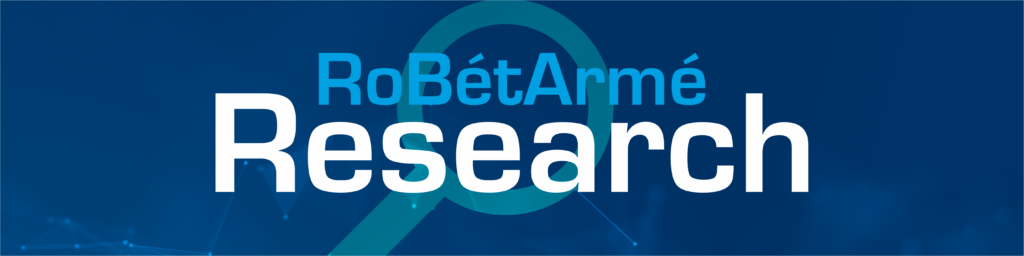
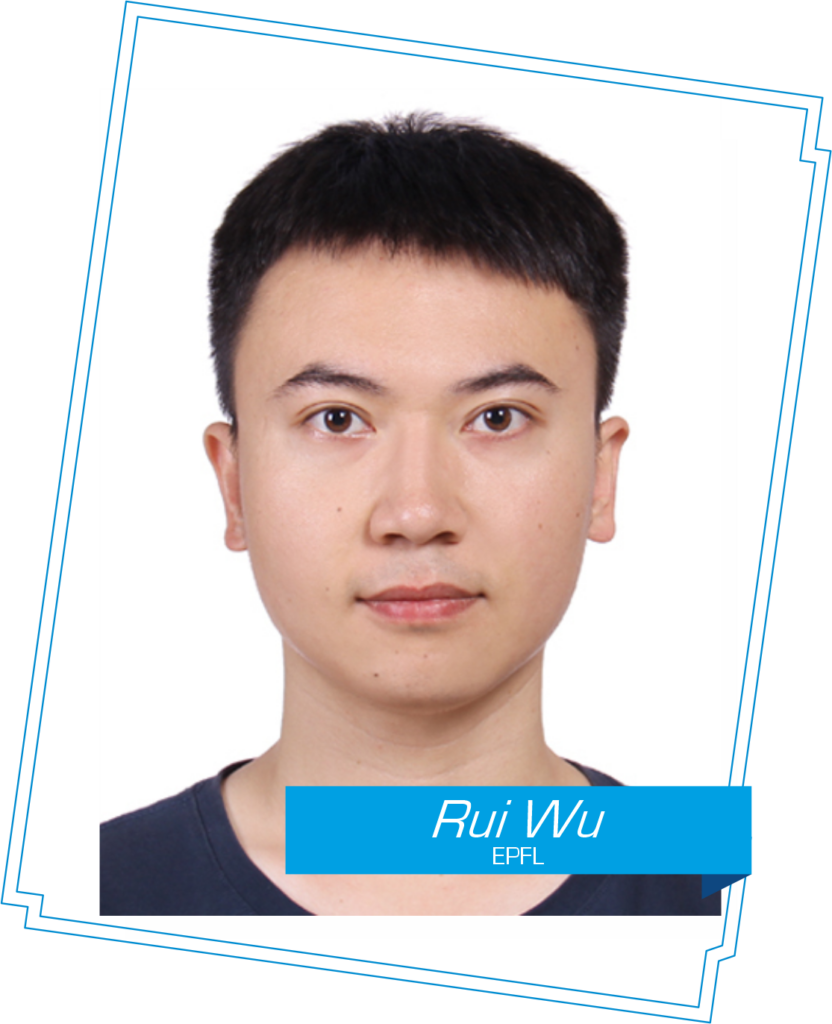
Can you tell us a bit about your background, and how it led you to become involved in the RoBétArmé project?
I come from China and am now working at EPFL to engage in postdoctoral research at the Learning Algorithms and Systems Laboratory (LASA). My PhD research focused on human-robot skill transfer and control. During this time, I developed several methods to enable robots to mimic human operational skills within a variable impedance control framework, successfully performing tasks such as cutting soft tissue and polishing uneven surfaces. The opportunity to integrate my research with real-world engineering challenges in the construction industry, and the prospect of seeing it applied successfully in the near future, is tremendously exciting for me.
Could you share some insights into your specific role and involvement in the project? What are your main responsibilities and contributions?
My main responsibility in our EPFL group is to address research challenges within our assigned work package. I concentrate on analyzing and extracting features from expert demonstration data to create models of human operational skills. These models are trained using real data and incorporate control mechanisms that enable robots to perform specific human tasks like shotcrete application and surface finishing. We successfully developed and implemented the first version of our skill transfer framework, conducting tests on two robots, KUKA IIWA and UR5, in our lab. The results demonstrate that our framework can effectively replicate expert skills, enabling robots to efficiently perform tasks in shotcrete application and surface finishing.
Research endeavors often come with challenges and obstacles. What are some of the main challenges you've encountered during your journey with RoBétArmé, and how have you managed to overcome them?
A primary challenge in our research has been the often messy and overlapping nature of human demonstrations, which complicates the direct application of machine learning algorithms to develop skill models. We segmented human operations into different levels and focused on feature extraction for individual levels. By incorporating frequency domain analysis methods, we successfully isolated two types of motion patterns in human operations and designed corresponding skill models. Another challenge is the instability that often occurs when robots attempt to replicate the dexterity and speed of human movements. To manage this, we adopted a dynamical systems approach in our model design to ensure motion stability. Additionally, we designed variable impedance algorithms in our controllers to stabilize the whole system.
Collaboration and networking are essential in research projects like RoBétArmé. Could you discuss some of your experiences collaborating with other researchers and institutions within the project?
We regularly meet with our partners to share updates on work progress and discuss issues and insights from our research. Through these interactions, we receive valuable feedback. For example, real workers demonstrate many details and skills in operation that are easy to overlook if we only check the demonstration data, and conversations with our industrial partners can give us a deeper understanding of the workers’ operations. Likewise, when exchanging ideas with our academic collaborators, we inspire each other and come up with more intriguing ideas. This continuous dialogue significantly enhances our project outcomes, effectively bridging practical and theoretical aspects.
Can you highlight any specific achievements or breakthroughs from your work within the project that you're particularly proud of?
Throughout the project, I have learned a lot and made significant contributions to research on human-robot skill transfer. As the first author, I submitted a conference paper and a journal article based on this work. Additionally, we successfully completed the first version of real robot demos for autonomous robotic shotcrete and surface finishing. These demos have been well-received and acknowledged by our collaborators, marking important milestones in our project.
Looking to the future, what are your hopes and aspirations for the impact of your research within RoBétArmé? How do you envision it contributing to advancements in your field and society as a whole?
I am eager to see our developed skill transfer algorithm framework integrate with the algorithms, sensors, and robots provided by our collaborators, forming a mature robotic system for use in construction engineering. This integration is both exciting and exhilarating. Our system will differ from current similar technologies by achieving more anthropomorphic operations and enhancing task performance through visual feedback. This will improve the precision, efficiency, and safety of autonomous spraying tasks. Under this system, workers and robots can collaborate, with workers relieved from strenuous physical labor. The presence of robots will transform the working environment and workload in the industry, enabling workers to focus more on handling complex and creative architectural details.